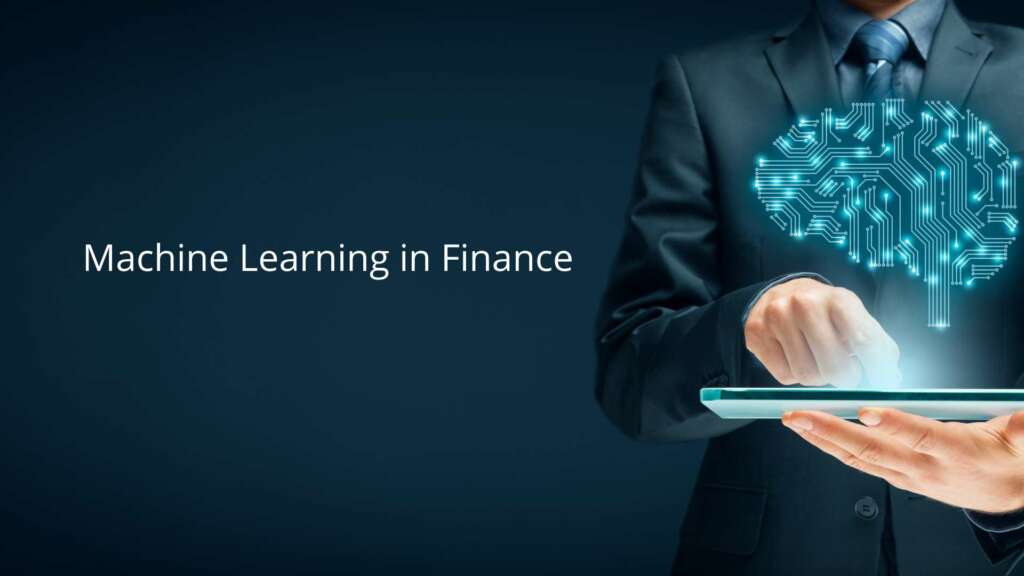
Machine learning has made major inroads into the finance industry recently. Let’s take a quick look at what this technology is, and why financial institutions need to embrace it. Machine learning is applying artificial intelligence to business operations.
The key to artificial intelligence in finance is that it learns by experience without being directly programmed. The goal is for it to be able to quickly learn what it needs to know, based on the results of past data that it has processed.
Many experts in finance agree that one of the biggest challenges for companies using machine learning in finance is figuring out how to effectively measure the progress of their new system. The difficulty with trying to measure the success or failure of machine learning in finance is that there are too many variables that could affect the results. One of the most important things is getting the machine to understand what its creators expect it to do. This may sound simple enough but there are some complicated algorithms involved.
Machine learning in finance also relies on more than just the accuracy of its predictions. It also needs to make sense. If it cannot understand what it is training for, then it will have trouble producing accurate predictions for future events.
To ensure that it is able to generate accurate predictions, business owners will need to use the right kind of machine learning in finance tools. The first of these tools is a program called a predictive model. A predictive model is a mathematical algorithm that helps businesses decide which trends to pay attention to. By understanding the factors that are behind the trend, businesses can plan appropriately for the upcoming market.
Predictive models have come under fire over the years because of their ability to produce predictions that do not always hold up when they are tested in real time. It’s important to remember that most of these models were designed to simply predict the behavior of an existing market. Although a predictive model may be able to accurately predict future market behavior, its results are based on previous data, which isn’t necessarily reflective of how the market will react when the model is used in real time.
Using a forecasting model in a good way is a good thing, but it does require a certain amount of skill and practice. There are a few different types of forecasting models that can be applied. Two of the most popular types are the RCTPA (Regression Coupled Modeling and the ARIMA (Area Independent Modeling). Some of the most popular forecasting models that are used today include forward and cross sectional models.
RCTPA is a forecasting model that is used by banks, brokers, and other financial institutions. The RCTPA uses historical data and past patterns to predict which trends are likely to occur in the future, while ARIMA uses past data and current trends to predict which trends are likely to occur in the future. While both models are useful, it is usually easier to implement the former since it uses the data from many different sources.
While this may seem obvious, predicting the future using a predictive model in finance requires a lot of intuition. It’s important to take the time to understand how the model works, so you can make better predictions in the future. As a general rule, the more information you have to work with, the more accurate the forecast will be. However, you can also use the model to get more information. When you do that, you are likely to come up with a more accurate forecast.
One of the most common problems that companies face when using the RCTPA model is figuring out the parameters that the model is based on. In order to avoid this problem, many use multiple sets of data to determine which parameters are the best for forecasting. Once you have the model running, you can then tweak some of the variables to see which ones are causing the model to produce better predictions.
Once the model has been put together, it will then need to be tested on actual data. To do that, it must be run repeatedly. This is where predictive testing is important.
While forecasting is an important part of the process, forecasting in finance does not have to be difficult. You can use a machine learning in finance tool to help test your predictions and to determine which methods are most likely to produce better predictions. If the machine learning in finance tool that you use gives you consistent results over time, then there’s no reason not to use it.